
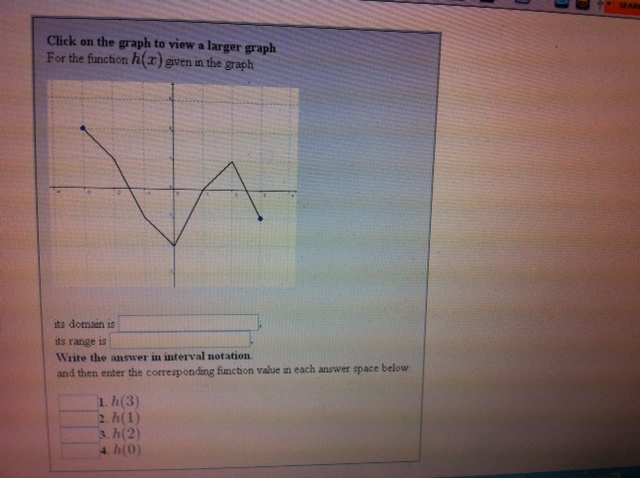
For example, in E&E, data are commonly presented in plots with means and standard errors or confidence intervals (Figure 1a), from which the user wants a mean and SD for each group presented.

Consequently, a large amount of additional data manipulation is required, that is different across plots types. Finally, digitising programs typically only provide the user with calibrated x, y coordinates from imported figures, and do not differentiate between common plot types that are used to present data. These data often subsequently need to be imported and edited using different software. Instead they require the user to manually import images and export the resulting digitised data into individual files one-by-one. Third, existing programs do not import sets of images for the user to systematically work through. This makes the downstream calculations laborious, as information has to be added later, typically using different software. Second, digitising programs do not allow the integration of metadata at the time of data extraction, such as experimental group or variable names and sample sizes. Having a tool that facilitates reproducibility in meta-analyses will increase transparency and aid in resolving the reproducibility crises seen in many fields (Parker et al., 2016 Peng, 2011 Peng, Dominici, & Zeger, 2006). First, although meta-analysis is an important tool in consolidating the data from multiple studies, many of the processes involved in data extraction are opaque and difficult to reproduce, making extending or replicating studies problematic. These data need to be manually extracted using digitising programs.Īlthough there are several tools that extract data from figures, including both standalone programs and r packages (reviewed in Table 1), these tools do not cater to the general needs of meta-analysts for four main reasons (here we focus on meta-analysis, although many points apply to extraction for comparative analysis). For example, 42% of the papers used in a recent meta-analysis presented some or all of the required data in figures (Noble et al., 2018). As well as being presented in the text or tables of research papers, descriptive statistics are frequently presented in figures. means, SD, sample sizes, correlation coefficients) extracted from primary literature. These techniques rely heavily on descriptive statistics (e.g. Meta-analyses are extremely important in providing a means of quantitatively synthesizing experimental and/or observational studies to evaluate empirical support for fundamental theory in E&E (Gurevitch, Koricheva, Nakagawa, & Stewart, 2018). The use of meta-analysis in E&E, in particular, is rapidly growing, not only in terms of the number of meta-analyses (in plant ecology alone the yearly number of published meta-analyses doubled from 2006 to 2012 ), but also in terms of their size (a recent meta-analysis, for example, included 6,440 effect sizes from 175 publications ). In the fields of ecology and evolution (E&E), these data are most commonly used for comparative and meta-analyses. In many different contexts, researchers make use of data presented in primary literature. We hope that by making the process of figure extraction more flexible and easy to conduct, it will improve the transparency and quality of meta-analyses in the future.
#Graphclick software
sample sizes, treatment/variable names) about experiments and is not integrated with other software to streamline analysis pipelines. Additionally, current software lacks an ability to incorporate important metadata (e.g. Extracting descriptive statistics from figures can be a slow process that is not easily reproducible.However, the exact values of such statistics are commonly hidden in figures. Research synthesis, such as comparative and meta-analyses, requires the extraction of effect sizes from primary literature, which are commonly calculated from descriptive statistics.
